Carnegie Mellon University and NVIDIA have teamed up to develop a new training technique that enables humanoid robots to perform complex athletic movements with unprecedented agility—from Cristiano Ronaldo’s signature mid-air spin celebration to Kobe Bryant’s fadeaway jump shot.
The framework, Aligning Simulation and Real Physics (ASAP), bridges a critical gap between simulation and reality by allowing humanoid robots to execute high-level athletic movements previously thought too complex for machines.
“Humanoid robots hold the potential for unparalleled versatility for performing human-like, whole-body skills,” the researchers noted in their paper. “However, achieving agile and coordinated whole-body motions remains a significant challenge due to the dynamics mismatch between simulation and the real world.”
ASAP tackles this challenge through a two-stage process.
First, it pre-trains motion tracking policies—the algorithmic rules that control the tracking—in simulation using human motion data. It then deploys these policies in the real world to collect data that helps bridge the gap between simulated and actual physics.
The result is a humanoid robot capable of replicating signature moves from sports legends, including Cristiano Ronaldo’s iconic “Siu” celebration (involving a 180-degree mid-air rotation), LeBron James’s “Silencer” celebration (featuring precise single-leg balancing) and Kobe Bryant’s fadeaway jump shot (which involves jumping and landing in one foot).
Beyond these fancy sports moves, the robot demonstrated other impressive feats like forward and side jumps of over 1 meter.
At first glance, the robots may still look clumsy, but this time, it’s mostly due to hardware limitations, as they have way less articulation than a human.
However, they have more dexterity than other robots thanks to the “delta action model”—a correction mechanism that compensates for the differences between simulated and real-world physics. “The delta action model effectively serves as a residual correction term for the dynamics gap.”
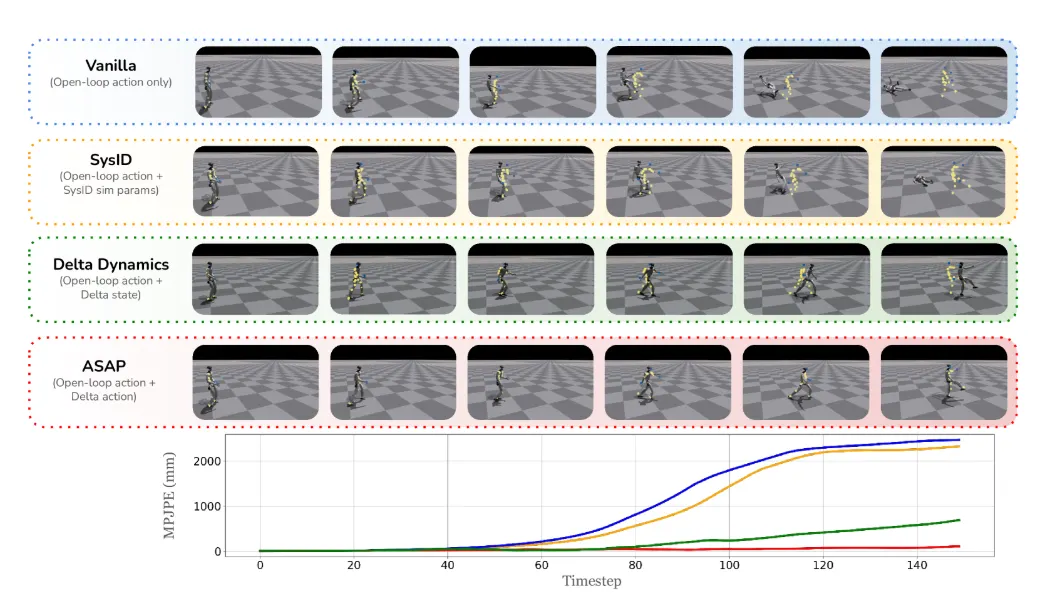
Using this approach, researchers reduced tracking errors by up to 52.7% compared to previous methods, enabling robots to perform complex movements that were previously impossible.
“Our approach significantly improves agility and whole-body coordination across various dynamic motions,” noted the researchers, who demonstrated the system’s effectiveness is “paving the way for versatile humanoid robots in real-world applications.”
Developing robots with this level of dexterity is particularly difficult and has been one of the most persistent challenges in robotics.
“For decades, we have envisioned humanoid robots achieving or even surpassing human-level agility. However, most prior work has primarily focused on locomotion, treating the legs as a means of mobility.” the researchers wrote.
ASAP, on the other hand, mimics the human body in pre-train and is able to adapt its knowledge to real-world scenarios after learning about it in simulations.
This way, the robot’s extremities behave like human extremities, being used for movement, balance, counterweight, expression, and more.
Achieving that is a lot harder than it seems. When we perform athletic—and even basic—moves, we’re actually orchestrating countless subtle adjustments in real-time, balancing multiple forces while compensating for changes in momentum and position.
Getting robots to replicate this has proven extraordinarily difficult.
Don’t believe us? try playing QWOP—a game in which you have to control 4 articulations to make an athlete run. Now, once you have spent hours mastering that game, think about how hard it would be to simultaneously manage the 21 basic articulations that ASAP handles—and then consider the human body has over 300 different joints.
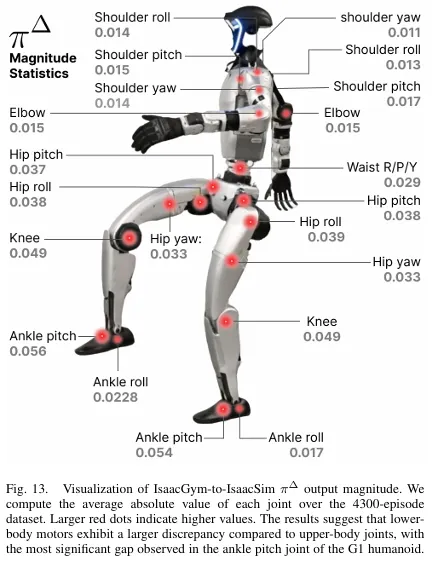
The field of humanoid robotics has been active in recent years, with companies and universities pouring more resources into R&D.
Tesla’s Optimus project, Figure AI’s recent humanoid robot announcement, and Boston Dynamics’ Atlas have all highlighted growing commercial interest in humanoid robots.
In the academic area, the University of Bristol and Stanford have also developed their own methods to teach models how to be more agile and increase their dexterity.
The team is focused on further developing ASAP
“Future directions could focus on developing damage-aware policy architectures to mitigate hardware risks,” they said, referring to how some models broke while trying to perform complex moves.
They also want to research “leveraging markerless pose estimation or onboard sensor fusion to reduce reliance on MoCap systems” and improve their adaptation techniques to achieve higher efficiency. How long before we have an all-robot World Cup?
Edited by Sebastian Sinclair and Josh Quittner
Generally Intelligent Newsletter
A weekly AI journey narrated by Gen, a generative AI model.